As The Size Of The Sample Increases, What Happens To The Shape Of The Distribution Of Sample Means?
half-dozen.2: The Sampling Distribution of the Sample Mean
- Page ID
- 570
Learning Objectives
- To learn what the sampling distribution of \(\overline{Ten}\) is when the sample size is large.
- To learn what the sampling distribution of \(\overline{X}\) is when the population is normal.
In Example 6.one.1, we synthetic the probability distribution of the sample hateful for samples of size ii drawn from the population of four rowers. The probability distribution is:
\[\begin{array}{c|c c c c c c c} \bar{10} & 152 & 154 & 156 & 158 & 160 & 162 & 164\\ \hline P(\bar{x}) &\dfrac{i}{sixteen} &\dfrac{two}{16} &\dfrac{three}{16} &\dfrac{4}{16} &\dfrac{three}{sixteen} &\dfrac{2}{16} &\dfrac{i}{16}\\ \end{array}\]
Effigy \(\PageIndex{one}\) shows a side-by-side comparison of a histogram for the original population and a histogram for this distribution. Whereas the distribution of the population is uniform, the sampling distribution of the mean has a shape budgeted the shape of the familiar bong curve. This phenomenon of the sampling distribution of the mean taking on a bell shape fifty-fifty though the population distribution is not bell-shaped happens in general. Here is a somewhat more realistic case.
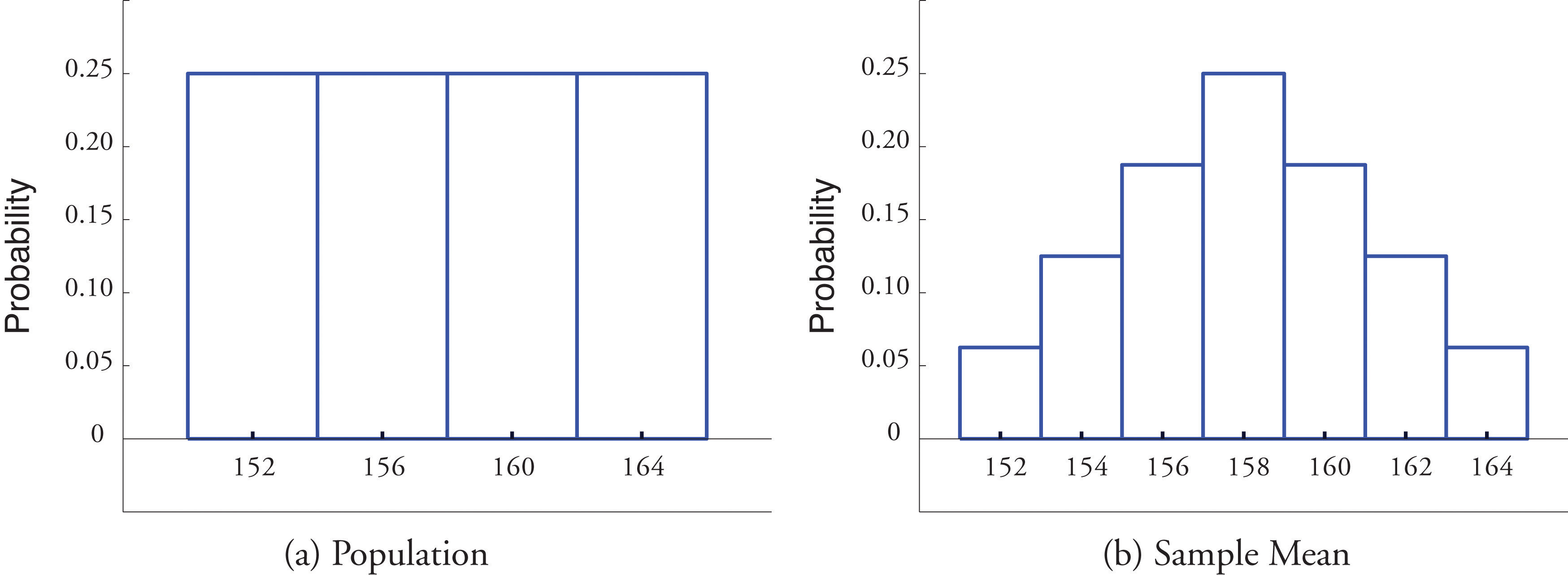
Suppose we take samples of size \(1\), \(5\), \(ten\), or \(xx\) from a population that consists entirely of the numbers \(0\) and \(ane\), half the population \(0\), half \(1\), and so that the population mean is \(0.5\). The sampling distributions are:
\(n = ane\):
\[\begin{assortment}{c|c c } \bar{x} & 0 & 1 \\ \hline P(\bar{x}) &0.5 &0.5 \\ \end{array} \nonumber\]
\(n = five\):
\[\begin{assortment}{c|c c c c c c} \bar{x} & 0 & 0.ii & 0.4 & 0.6 & 0.8 & i \\ \hline P(\bar{x}) &0.03 &0.16 &0.31 &0.31 &0.16 &0.03 \\ \end{array} \nonumber\]
\(north = ten\):
\[\brainstorm{array}{c|c c c c c c c c c c c} \bar{x} & 0 & 0.1 & 0.2 & 0.three & 0.four & 0.5 & 0.6 & 0.7 & 0.8 & 0.ix & 1 \\ \hline P(\bar{x}) &0.00 &0.01 &0.04 &0.12 &0.21 &0.25 &0.21 &0.12 &0.04 &0.01 &0.00 \\ \end{assortment} \nonumber\]
\(n = 20\):
\[\begin{array}{c|c c c c c c c c c c c} \bar{ten} & 0 & 0.05 & 0.10 & 0.15 & 0.20 & 0.25 & 0.30 & 0.35 & 0.xl & 0.45 & 0.50 \\ \hline P(\bar{x}) &0.00 &0.00 &0.00 &0.00 &0.00 &0.01 &0.04 &0.07 &0.12 &0.16 &0.18 \\ \end{array} \nonumber\]
and
\[\begin{assortment}{c|c c c c c c c c c c } \bar{x} & 0.55 & 0.60 & 0.65 & 0.70 & 0.75 & 0.80 & 0.85 & 0.90 & 0.95 & ane \\ \hline P(\bar{x}) &0.16 &0.12 &0.07 &0.04 &0.01 &0.00 &0.00 &0.00 &0.00 &0.00 \\ \end{array} \nonumber\]
Histograms illustrating these distributions are shown in Figure \(\PageIndex{2}\).
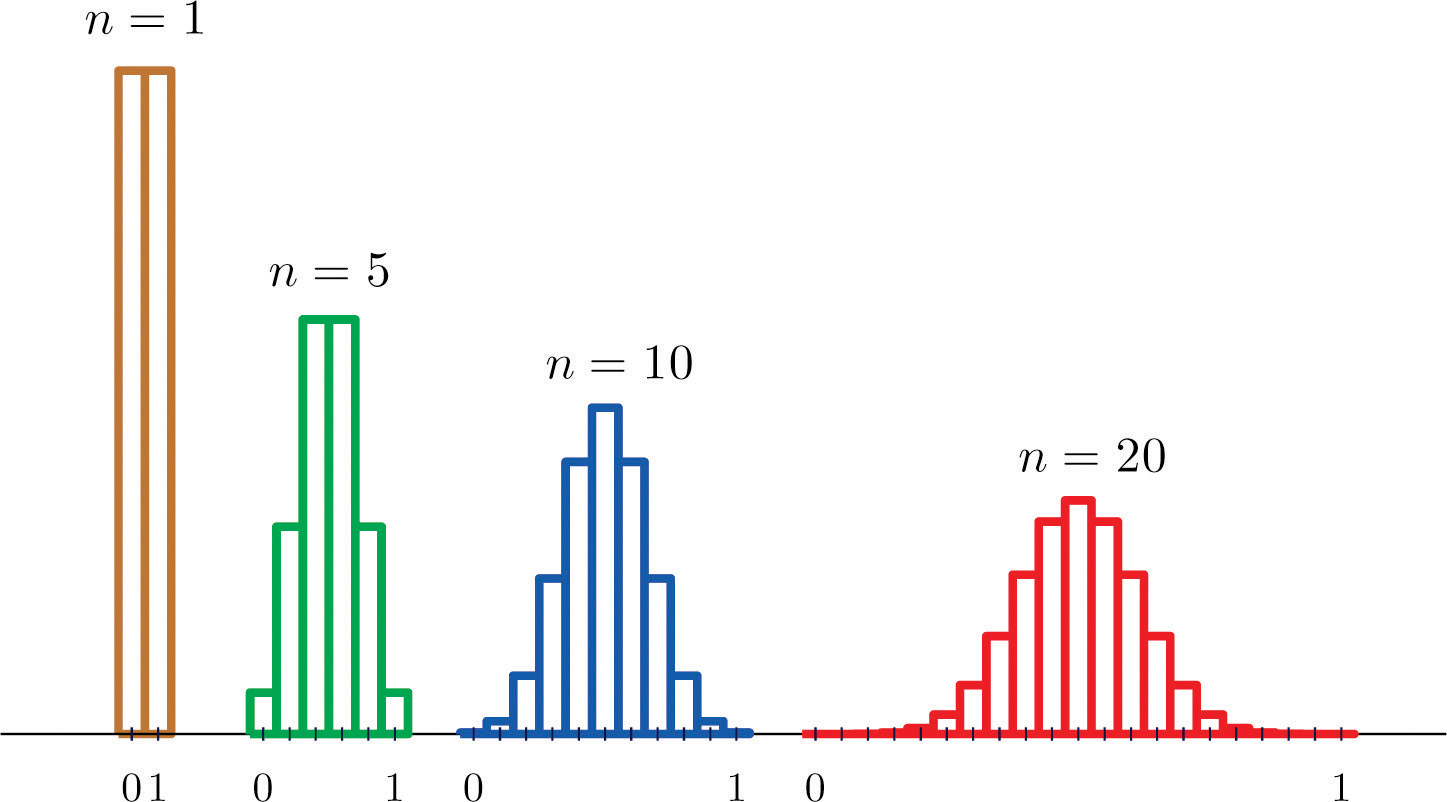
Equally \(n\) increases the sampling distribution of \(\overline{Ten}\) evolves in an interesting manner: the probabilities on the lower and the upper ends shrink and the probabilities in the centre get larger in relation to them. If we were to continue to increase \(n\) then the shape of the sampling distribution would become smoother and more bell-shaped.
What nosotros are seeing in these examples does non depend on the item population distributions involved. In full general, i may showtime with any distribution and the sampling distribution of the sample mean will increasingly resemble the bell-shaped normal curve as the sample size increases. This is the content of the Central Limit Theorem.
The Central Limit Theorem
For samples of size \(thirty\) or more than, the sample mean is approximately normally distributed, with hateful \(\mu _{\overline{X}}=\mu\) and standard difference \(\sigma _{\overline{X}}=\dfrac{\sigma }{\sqrt{n}}\), where \(due north\) is the sample size. The larger the sample size, the better the approximation. The Fundamental Limit Theorem is illustrated for several common population distributions in Effigy \(\PageIndex{iii}\).
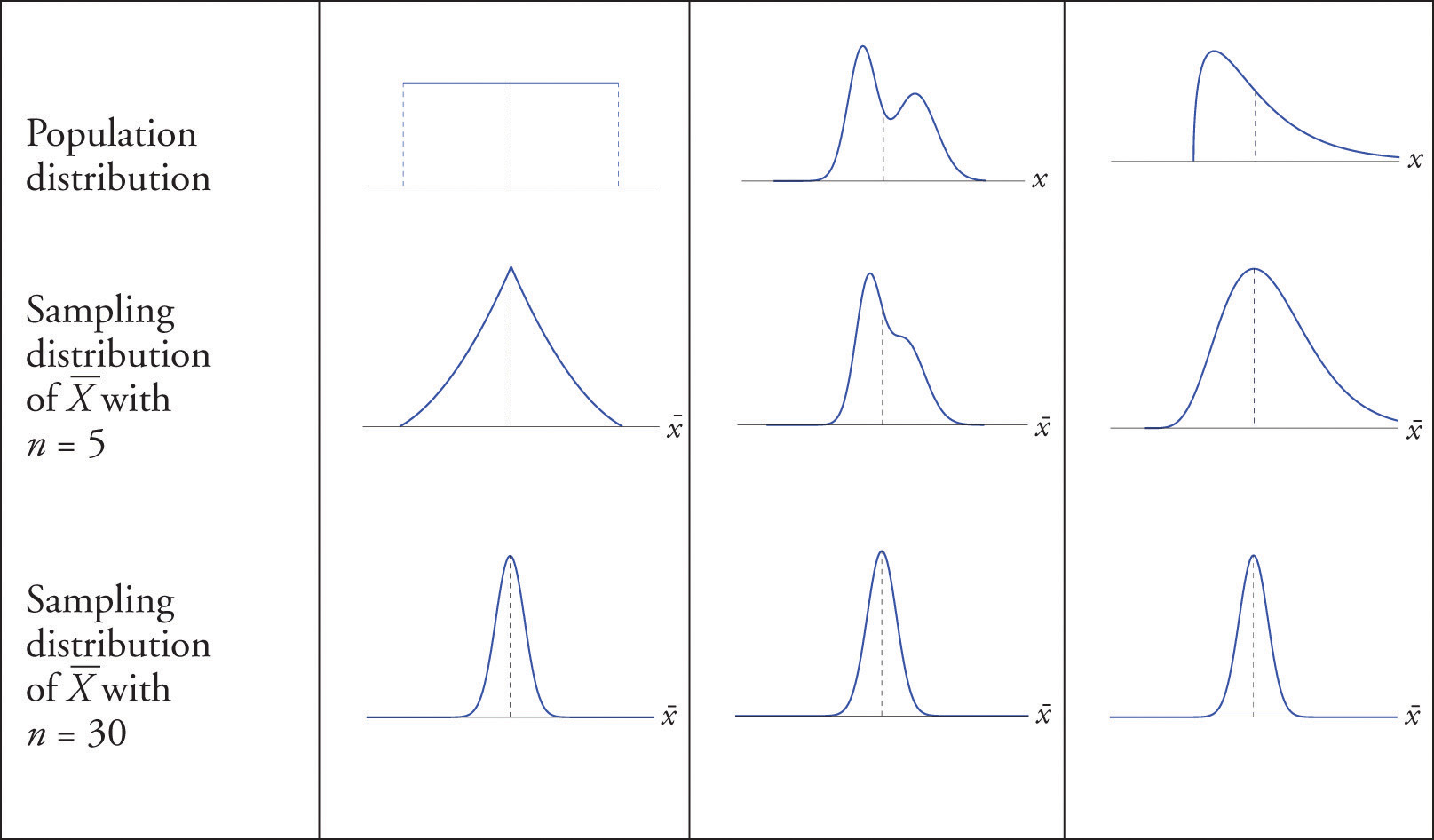
The dashed vertical lines in the figures locate the population mean. Regardless of the distribution of the population, equally the sample size is increased the shape of the sampling distribution of the sample hateful becomes increasingly bell-shaped, centered on the population mean. Typically by the time the sample size is \(xxx\) the distribution of the sample mean is practically the same as a normal distribution.
The importance of the Central Limit Theorem is that it allows us to make probability statements about the sample mean, specifically in relation to its value in comparison to the population mean, as nosotros will see in the examples. Only to use the result properly we must first realize that in that location are two split random variables (and therefore two probability distributions) at play:
- \(X\), the measurement of a single element selected at random from the population; the distribution of \(10\) is the distribution of the population, with mean the population mean \(\mu\) and standard deviation the population standard deviation \(\sigma\);
- \(\overline{Ten}\), the mean of the measurements in a sample of size \(n\); the distribution of \(\overline{10}\) is its sampling distribution, with mean \(\mu _{\overline{X}}=\mu\) and standard difference \(\sigma _{\overline{X}}=\dfrac{\sigma }{\sqrt{n}}\).
Example \(\PageIndex{1}\)
Allow \(\overline{10}\) exist the hateful of a random sample of size \(50\) drawn from a population with hateful \(112\) and standard deviation \(40\).
- Find the mean and standard deviation of \(\overline{10}\).
- Discover the probability that \(\overline{X}\) assumes a value between \(110\) and \(114\).
- Observe the probability that \(\overline{X}\) assumes a value greater than \(113\).
Solution:
- By the formulas in the previous section \[\mu _{\overline{Ten}}=\mu=112 \nonumber\] and \[ \sigma_{\overline{X}}=\dfrac{\sigma}{\sqrt{n}}=\dfrac{40} {\sqrt{l}}=v.65685 \nonumber\]
- Since the sample size is at to the lowest degree \(30\), the Primal Limit Theorem applies: \(\overline{Ten}\) is approximately normally distributed. We compute probabilities using Figure 5.3.1 in the usual style, just existence conscientious to utilize \(\sigma _{\overline{10}}\) and not \(\sigma\) when we standardize:
\[\begin{marshal*} P(110<\overline{X}<114)&= P\left ( \dfrac{110-\mu _{\overline{X}}}{\sigma _{\overline{X}}} <Z<\dfrac{114-\mu _{\overline{X}}}{\sigma _{\overline{X}}}\right )\\[4pt] &= P\left ( \dfrac{110-112}{5.65685} <Z<\dfrac{114-112}{5.65685}\right )\\[4pt] &= P(-0.35<Z<0.35)\\[4pt] &= 0.6368-0.3632\\[4pt] &= 0.2736 \cease{marshal*}\]
- Similarly
\[\brainstorm{align*} P(\overline{X}> 113)&= P\left ( Z>\dfrac{113-\mu _{\overline{Ten}}}{\sigma _{\overline{X}}}\correct )\\[4pt] &= P\left ( Z>\dfrac{113-112}{v.65685}\right )\\[4pt] &= P(Z>0.18)\\[4pt] &= ane-P(Z<0.xviii)\\[4pt] &= ane-0.5714\\[4pt] &= 0.4286 \end{align*}\]
Note that if in the above example we had been asked to compute the probability that the value of a unmarried randomly selected element of the population exceeds \(113\), that is, to compute the number \(P(X>113)\), we would non have been able to practise and then, since nosotros exercise non know the distribution of \(Ten\), but merely that its mean is \(112\) and its standard deviation is \(forty\). By contrast we could compute \(P(\overline{10}>113)\) even without complete cognition of the distribution of \(Ten\) considering the Central Limit Theorem guarantees that \(\overline{Ten}\) is approximately normal.
Case \(\PageIndex{two}\)
The numerical population of grade point averages at a college has hateful \(2.61\) and standard divergence \(0.5\). If a random sample of size \(100\) is taken from the population, what is the probability that the sample mean will be between \(2.51\) and \(2.71\)?
Solution:
The sample hateful \(\overline{X}\) has mean \(\mu _{\overline{10}}=\mu =2.61\) and standard deviation \(\sigma _{\overline{10}}=\dfrac{\sigma }{\sqrt{n}}=\dfrac{0.five}{ten}=0.05\), and so
\[\begin{align*} P(2.51<\overline{10}<2.71)&= P\left ( \dfrac{two.51-\mu _{\overline{X}}}{\sigma _{\overline{Ten}}} <Z<\dfrac{ii.71-\mu _{\overline{10}}}{\sigma _{\overline{Ten}}}\right )\\[4pt] &= P\left ( \dfrac{2.51-2.61}{0.05} <Z<\dfrac{2.71-2.61}{0.05}\correct )\\[4pt] &= P(-ii<Z<2)\\[4pt] &= P(Z<2)-P(Z<-2)\\[4pt] &= 0.9772-0.0228\\[4pt] &= 0.9544 \end{align*}\]
Commonly Distributed Populations
The Primal Limit Theorem says that no thing what the distribution of the population is, as long as the sample is "large," pregnant of size \(xxx\) or more, the sample mean is approximately normally distributed. If the population is normal to brainstorm with then the sample mean also has a normal distribution, regardless of the sample size.
For samples of any size drawn from a commonly distributed population, the sample mean is normally distributed, with mean \(μ_X=μ\) and standard divergence \(σ_X =σ/\sqrt{n}\), where \(n\) is the sample size.
The consequence of increasing the sample size is shown in Effigy \(\PageIndex{four}\).
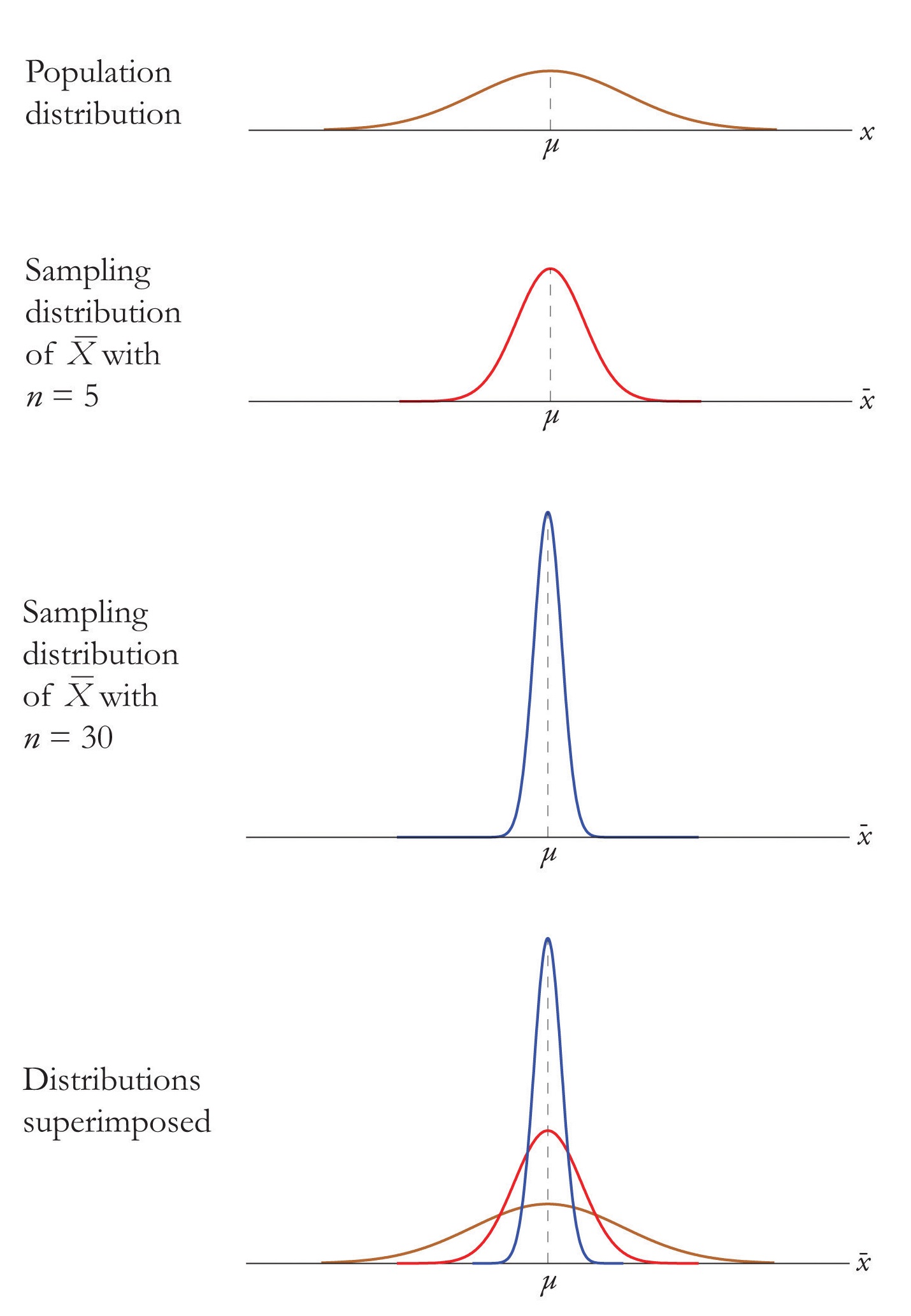
Instance \(\PageIndex{3}\)
A prototype automotive tire has a design life of \(38,500\) miles with a standard deviation of \(ii,500\) miles. Five such tires are manufactured and tested. On the assumption that the bodily population mean is \(38,500\) miles and the actual population standard deviation is \(two,500\) miles, notice the probability that the sample mean will be less than \(36,000\) miles. Assume that the distribution of lifetimes of such tires is normal.
Solution:
For simplicity we utilise units of thousands of miles. Then the sample mean \(\overline{X}\) has mean \(\mu _{\overline{X}}=\mu =38.5\) and standard deviation \(\sigma _{\overline{10}}=\dfrac{\sigma }{\sqrt{n}}=\dfrac{two.five}{\sqrt{v}}=i.11803\). Since the population is normally distributed, so is \(\overline{10}\), hence
\[\begin{align*} P(\overline{X}<36)&= P\left ( Z<\dfrac{36-\mu _{\overline{X}}}{\sigma _{\overline{X}}}\right )\\[4pt] &= P\left ( Z<\dfrac{36-38.five}{1.11803}\correct )\\[4pt] &= P(Z<-2.24)\\[4pt] &= 0.0125 \end{align*}\]
That is, if the tires perform every bit designed, there is only almost a \(one.25\%\) hazard that the boilerplate of a sample of this size would exist and then low.
Example \(\PageIndex{4}\)
An automobile bombardment manufacturer claims that its midgrade battery has a mean life of \(50\) months with a standard deviation of \(6\) months. Suppose the distribution of battery lives of this item brand is approximately normal.
- On the assumption that the manufacturer'southward claims are true, find the probability that a randomly selected battery of this type will last less than \(48\) months.
- On the same supposition, observe the probability that the hateful of a random sample of \(36\) such batteries will exist less than \(48\) months.
Solution:
- Since the population is known to have a normal distribution
\[\begin{align*} P(X<48)&= P\left ( Z<\dfrac{48-\mu }{\sigma }\correct )\\[4pt] &= P\left ( Z<\dfrac{48-l}{6}\right )\\[4pt] &= P(Z<-0.33)\\[4pt] &= 0.3707 \end{align*}\]
- The sample mean has mean \(\mu _{\overline{10}}=\mu =50\) and standard deviation \(\sigma _{\overline{X}}=\dfrac{\sigma }{\sqrt{n}}=\dfrac{half-dozen}{\sqrt{36}}=i\). Thus
\[\begin{marshal*} P(\overline{X}<48)&= P\left ( Z<\dfrac{48-\mu _{\overline{X}}}{\sigma _{\overline{Ten}}}\right )\\[4pt] &= P\left ( Z<\dfrac{48-50}{1}\right )\\[4pt] &= P(Z<-2)\\[4pt] &= 0.0228 \terminate{marshal*}\]
Key Takeaway
- When the sample size is at least \(30\) the sample hateful is unremarkably distributed.
- When the population is normal the sample mean is normally distributed regardless of the sample size.
As The Size Of The Sample Increases, What Happens To The Shape Of The Distribution Of Sample Means?,
Source: https://stats.libretexts.org/Bookshelves/Introductory_Statistics/Book%3A_Introductory_Statistics_(Shafer_and_Zhang)/06%3A_Sampling_Distributions/6.02%3A_The_Sampling_Distribution_of_the_Sample_Mean
Posted by: jarmansivionts35.blogspot.com
0 Response to "As The Size Of The Sample Increases, What Happens To The Shape Of The Distribution Of Sample Means?"
Post a Comment